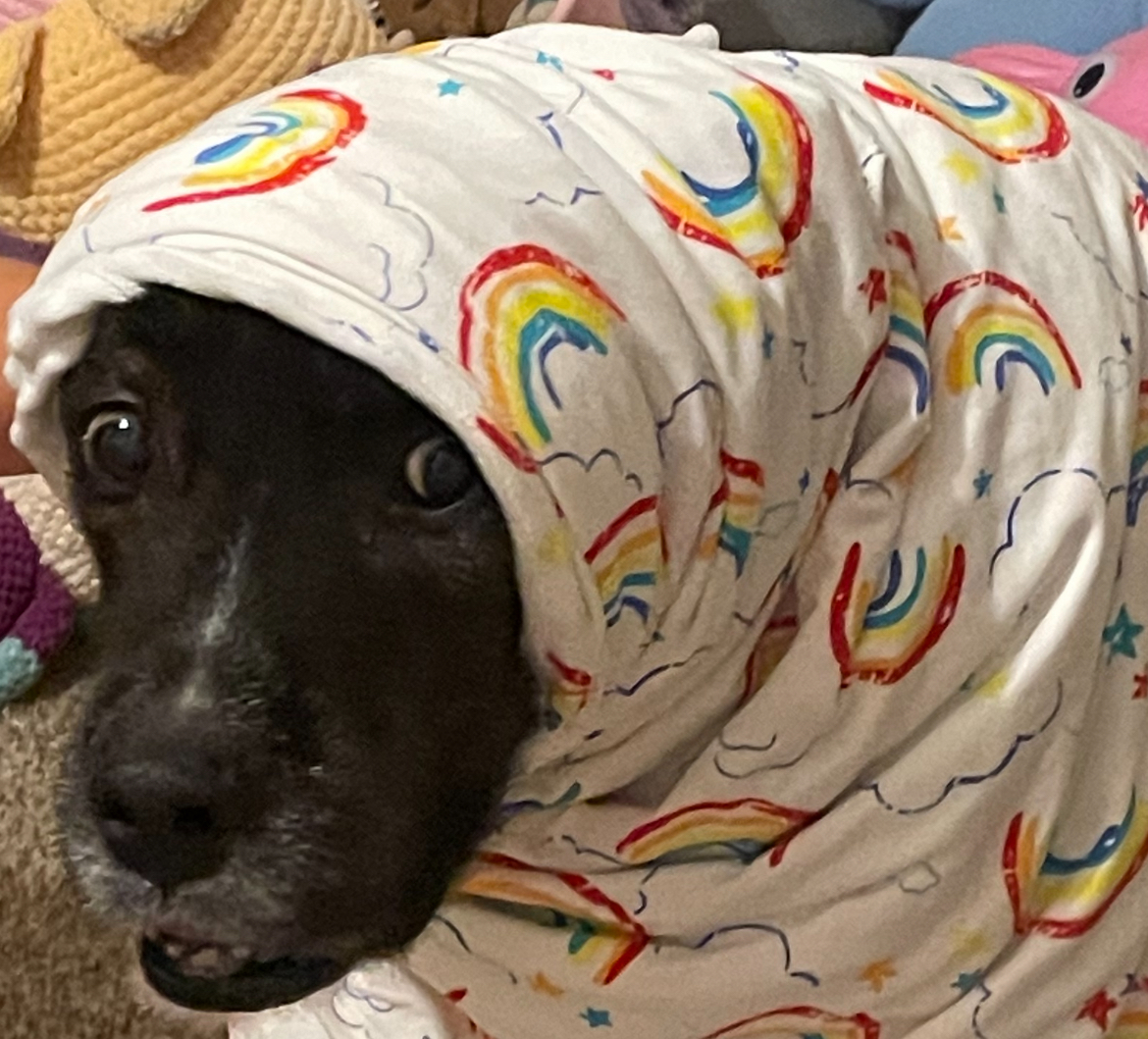
tom mcandrew (pi)
Principal Investigator; applied math, stats, human judgment, forecasting, infectious diseases
Principal Investigator; applied math, stats, human judgment, forecasting, infectious diseases
We presented 3000 humans with simulated surveillance data about the number of incident hospitalizations from a current and two past seasons, and asked that they predict the peak time and intensity of the underlying epidemic
We propose a novel Cluster-Aggregate-Pool or `CAP’ ensemble algorithm that first clusters together individual forecasts, aggregates individual models that belong to the same cluster into a single forecast (called a cluster forecast), and then pools together cluster forecasts via a linear pool.
These results suggest that a human judgment forecasting platform can quickly generate probabilistic predictions for targets of public health importance
Our work shows that aggregated human judgment forecasts for infectious agents are timely, accurate, and adaptable, and can be used as a tool to aid public health decision making during outbreaks.
Needing no data at the beginning of an epidemic, an adaptive ensemble can quickly train and forecast an outbreak, providing a practical tool to public health officials looking for a forecast to conform to unique features of a specific season.
This work highlights the importance that an expert linear pool could play in flexibly assessing a wide array of risks early in future emerging outbreaks, especially in settings where available data cannot yet support data-driven computational modeling.
Download Paper Abstract Forecasts support decision making in a variety of applications. Statistical models can produce accurate forecasts given abundant training data, but when data is sparse or rapidly changing, statistical models may not be able to make accurate predictions. Expert judgmental forecasts—models that combine expert-generated predictions into a single forecast—can make predictions when training data is limited by relying on human intuition. Researchers have proposed a wide array of algorithms to combine expert predictions into a single forecast, but there is no consensus on an optimal aggregation model....
Past lab members